Thanks to new digital tools, new methods of research are emerging. Ecologists, for example, use camera traps with motion triggers to observe animals in the wild. Scientists increasingly rely on machine learning in this context to analyze the large data volumes generated. The video installation Triggered by Motion documents this process.
Until the 1990s, there were only two options when studying wildlife: either individual animals were captured, studied, tagged, and released, or researchers observed them in the field. In contrast, camera traps allow observation without resorting to invasive means like collars or ear tags, while also avoiding influencing the behavior of the animal populations in question.
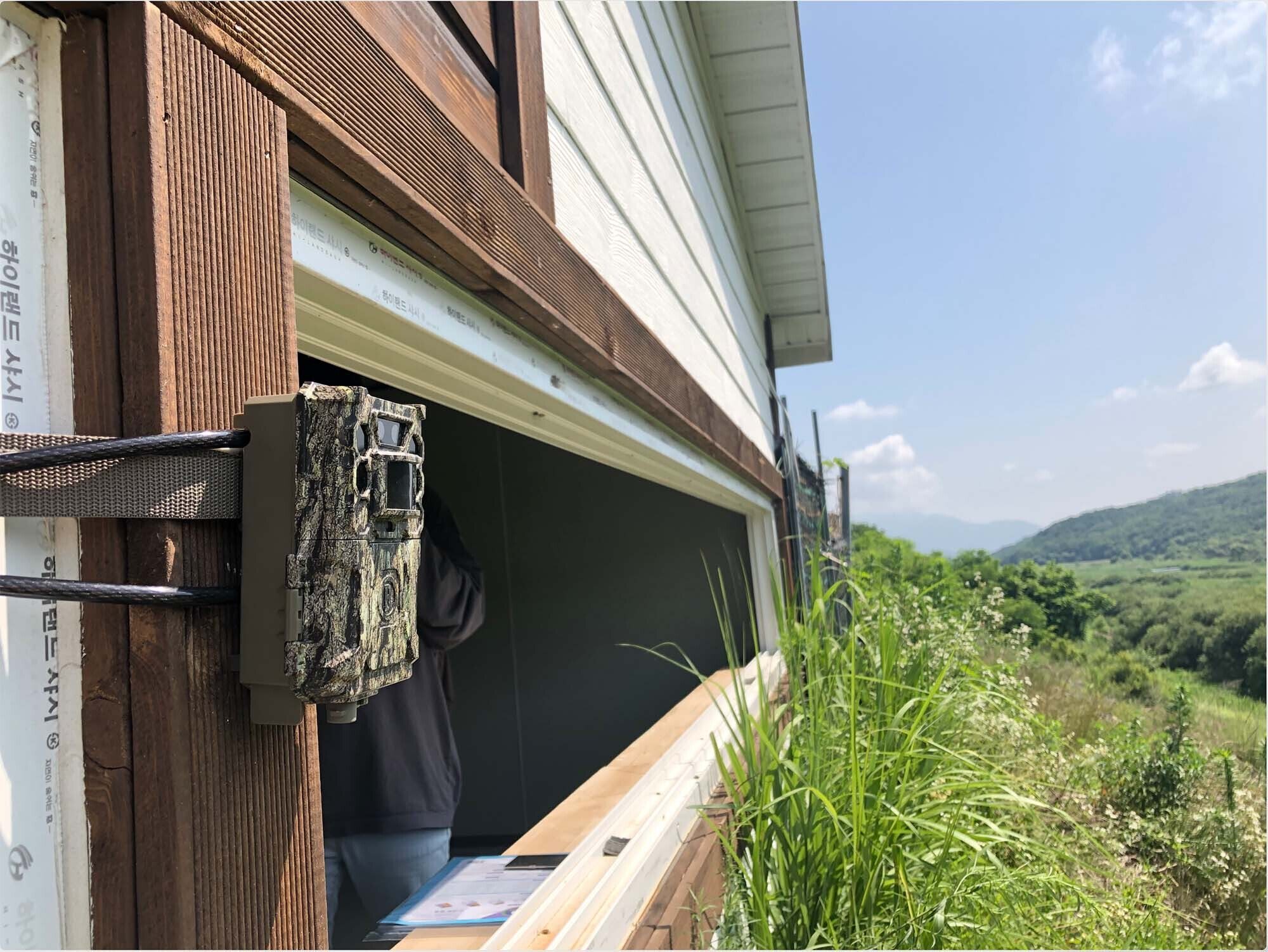
Discreet and compact: Camera trap at the Hantan River Crane Observatory. (Image: Centre for Anthropocene Studies, KAIST)
However, these cameras generate huge amounts of data that are classified manually, which is very time-consuming. Artificial intelligence can give a helping hand here. Intensive research is currently underway into methods that deploy machine learning to speed up image evaluation. In addition to AI for particular species, such as cranes (South Korea, demilitarized zone), wild dogs (Botswana, Okavango Delta) or ibex (Italy, Gran Paradiso National Park), this also involves cross-project algorithms designed to be applicable to a growing number of animal species.
That sets the bar high for researchers, and interdisciplinary collaboration is usually required. In this context, the Triggered by Motion video installation functions as a networking forum. Through online meetings, lectures, webinars, and lively debates, work on the installation has brought together researchers from around the world in fields that include wildlife research, conservation, and image/video data analysis. In the process, intriguing new ideas and initiatives are generated. Triggered by Motion now aims to bring this process to a broader audience. After all, although the future of digital conservation looks promising, conservation projects only work if they enjoy support beyond expert circles.
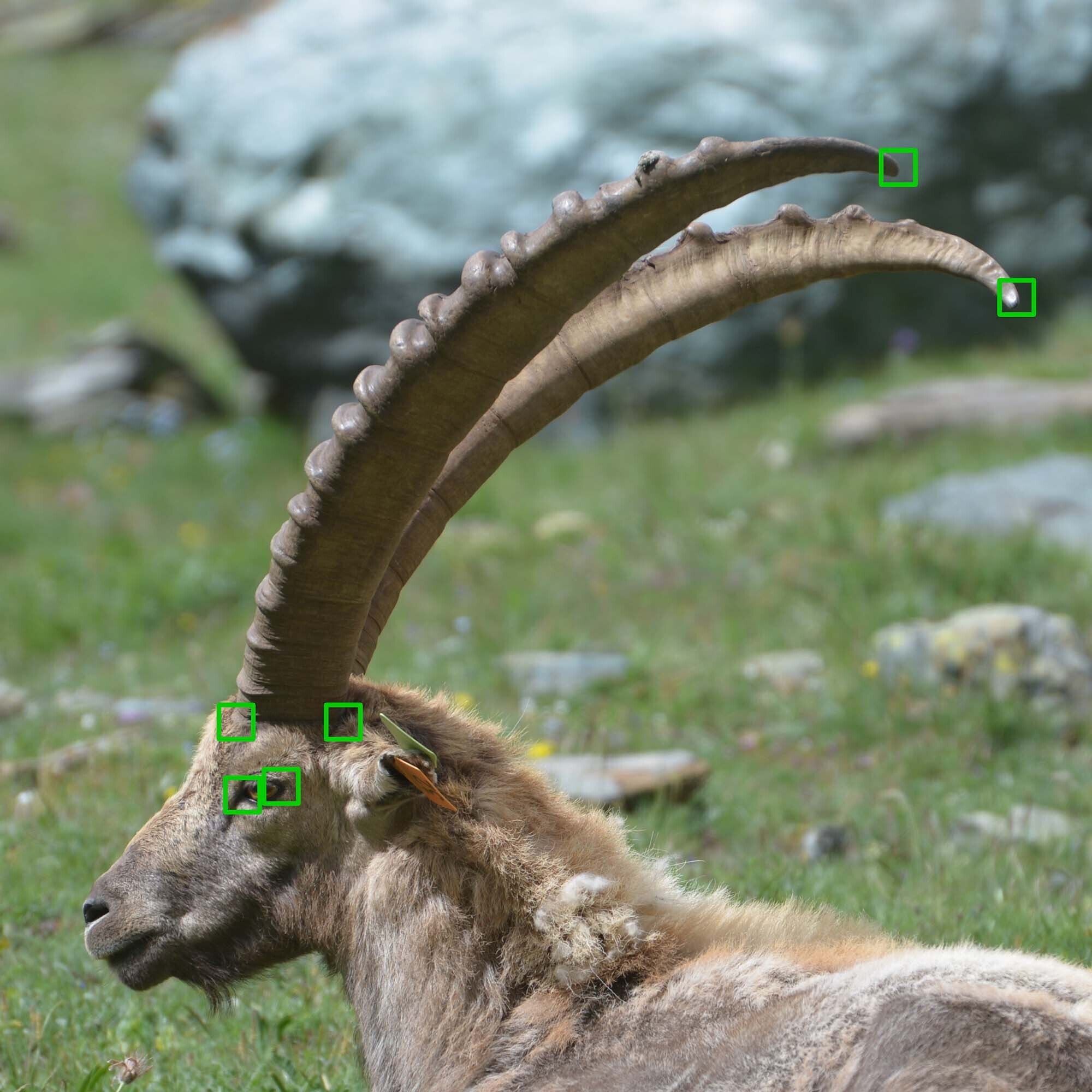
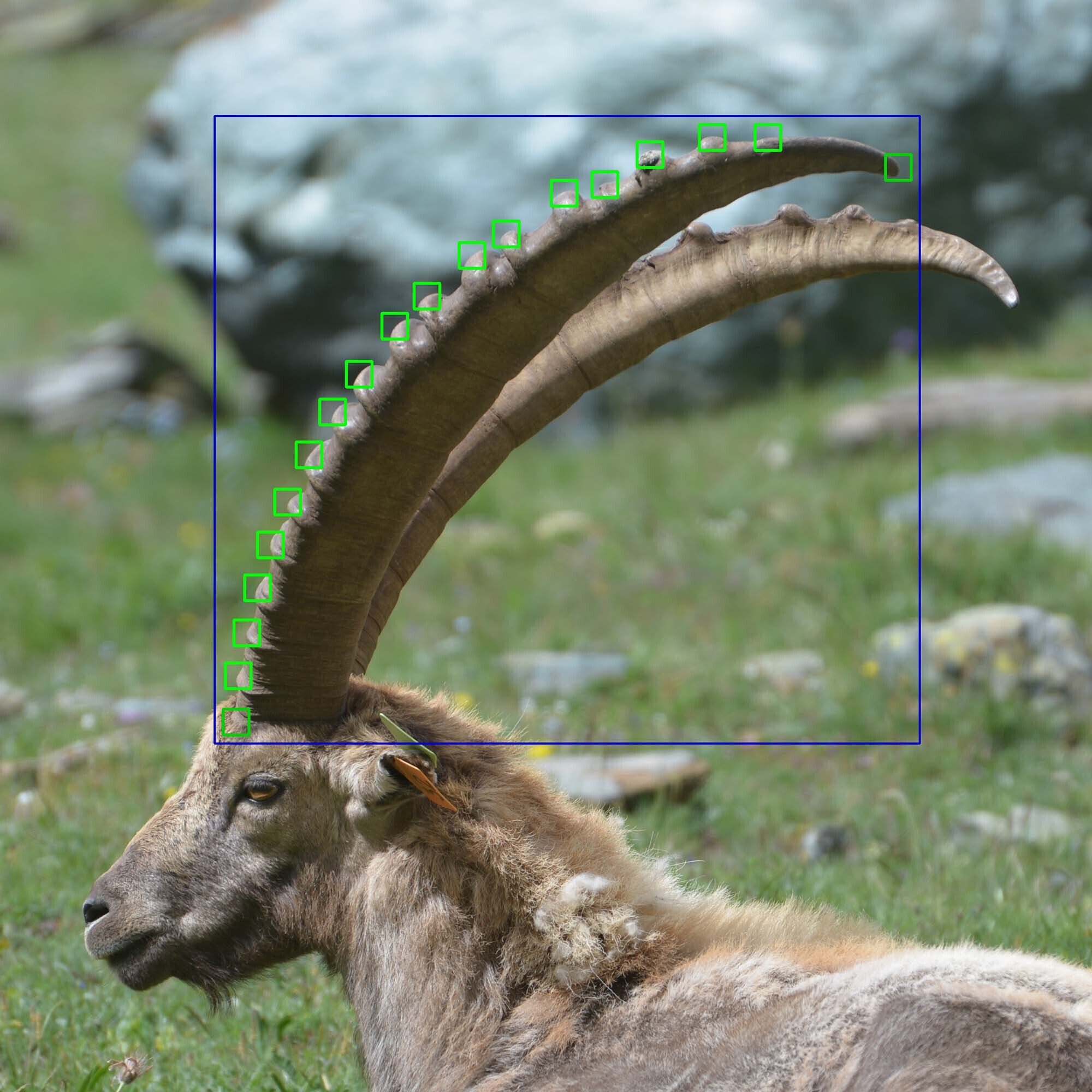
In the Ibex Project, individual ibex are identified using what are known as landmarks. (Image: Alice Brambilla)
For the installation, video data from 21 conservation projects worldwide were collected for an entire year, synchronized, and turned into 20-minute films. As a result, the films in the walk-in pavilion reveal global rhythms, alternating between day, night, and the changing seasons.
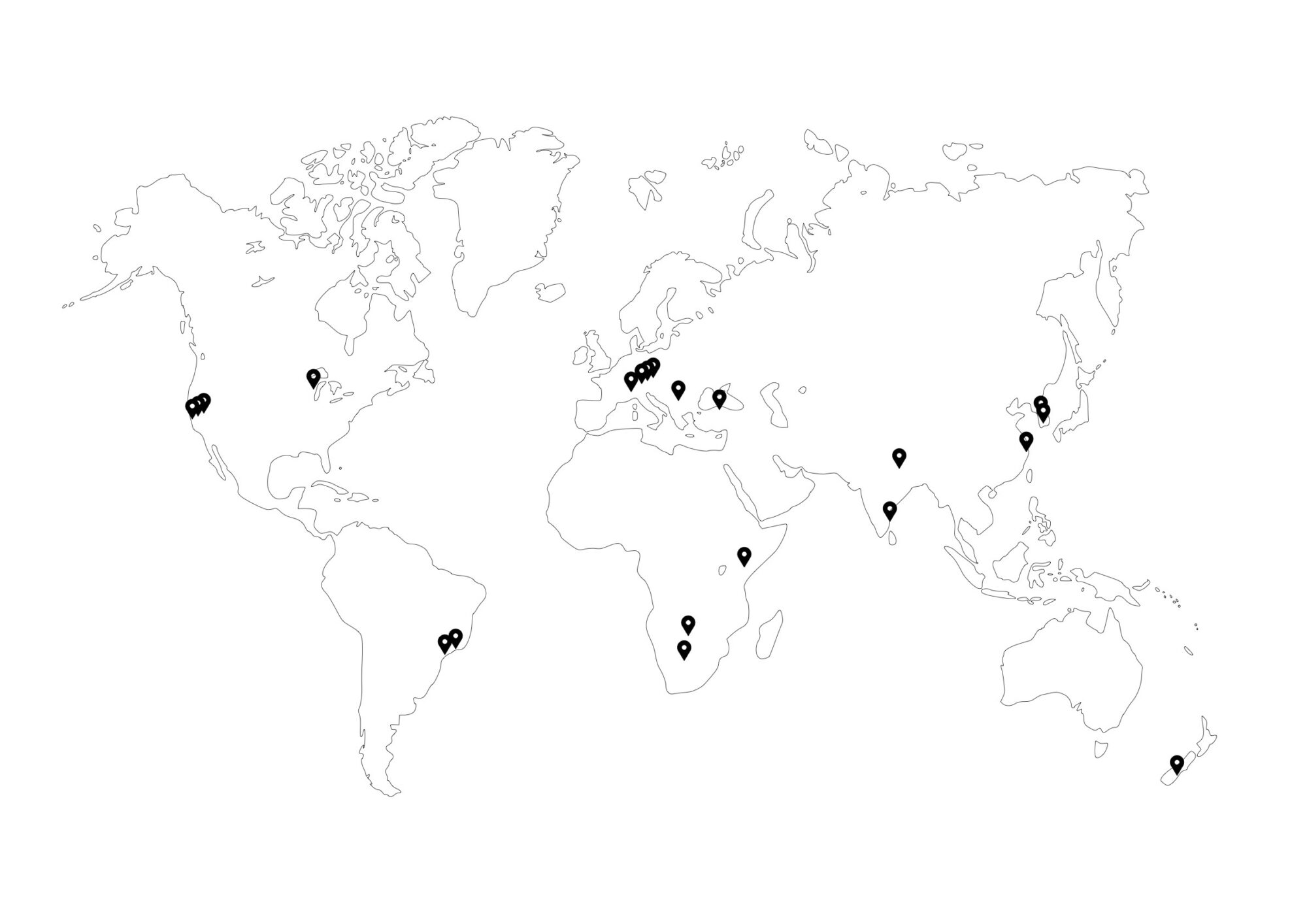
You can find more information about the camera locations in our interactive map.
The pavilion’s parametric design encompasses 1,547 different components. The screens inside are arranged to reflect the geographic distribution of the camera locations. They form the parameters for the pavilion’s design, which was conceived by Dino Rossi in Grasshopper, an algorithm-based visual programming language. Swiss company Impact Acoustic, which combines high standards of functionality and design with a consistent commitment to sustainability, took charge of producing the components: Triggered by Motion’s pavilion is made from a special sound-absorbing material, created by upcycling around 33,000 disposable plastic bottles.
A glimpse behind the scenes: build-up and design of the pavilion. (© Frank Brüderli and Dino Rossi)
Machine learning and in particular artificial intelligence are terms that crop up frequently nowadays. However, explaining them is not so easy. Determining what constitutes intelligence is a philosophical question that makes artificial intelligence correspondingly tricky to define. Machine learning, on the other hand, is easier to explain: it refers to computer programs or algorithms that constantly improve their performance through experience, i.e., programs or algorithms that learn. Machine learning algorithms can be viewed as a particular form of artificial intelligence, as they imitate the cognitive abilities of the human brain.
Both terms were coined in the 1950s, when digital computers with steadily growing processing power became widespread in research institutions. In the 1980s, the technology began to filter through into more and more disciplines. For some years now, these methods have also had an impact on ecological studies. The open source platform LINC (Lion Identification Network of Collaborators) is a good example of how machine learning can be applied in ecology nowadays – it is used, for instance, by Dominic Maringa’s team in Kenya, which is involved in the Triggered by Motion project.
Relative frequency of the terms “machine learning” and “artificial intelligence” since 1970. The graph also shows the first AI boom in the 1980s. (figure: Google Ngram Viewer)
LINC aims to facilitate study and conservation of lion populations across Africa by promoting cooperation between institutions and across national borders. This is urgently needed, as the African lion has lost 42 percent of its habitat since the turn of the millennium. The various populations have consequently become increasingly isolated from each another. Data on the lions’ migratory behavior is hugely important to maintain genetic diversity among them and prevent populations from dying out due to inbreeding. The data collected via LINC will provide insights into the areas where the lions spend most of their time, as well as on when and how they migrate to other areas.
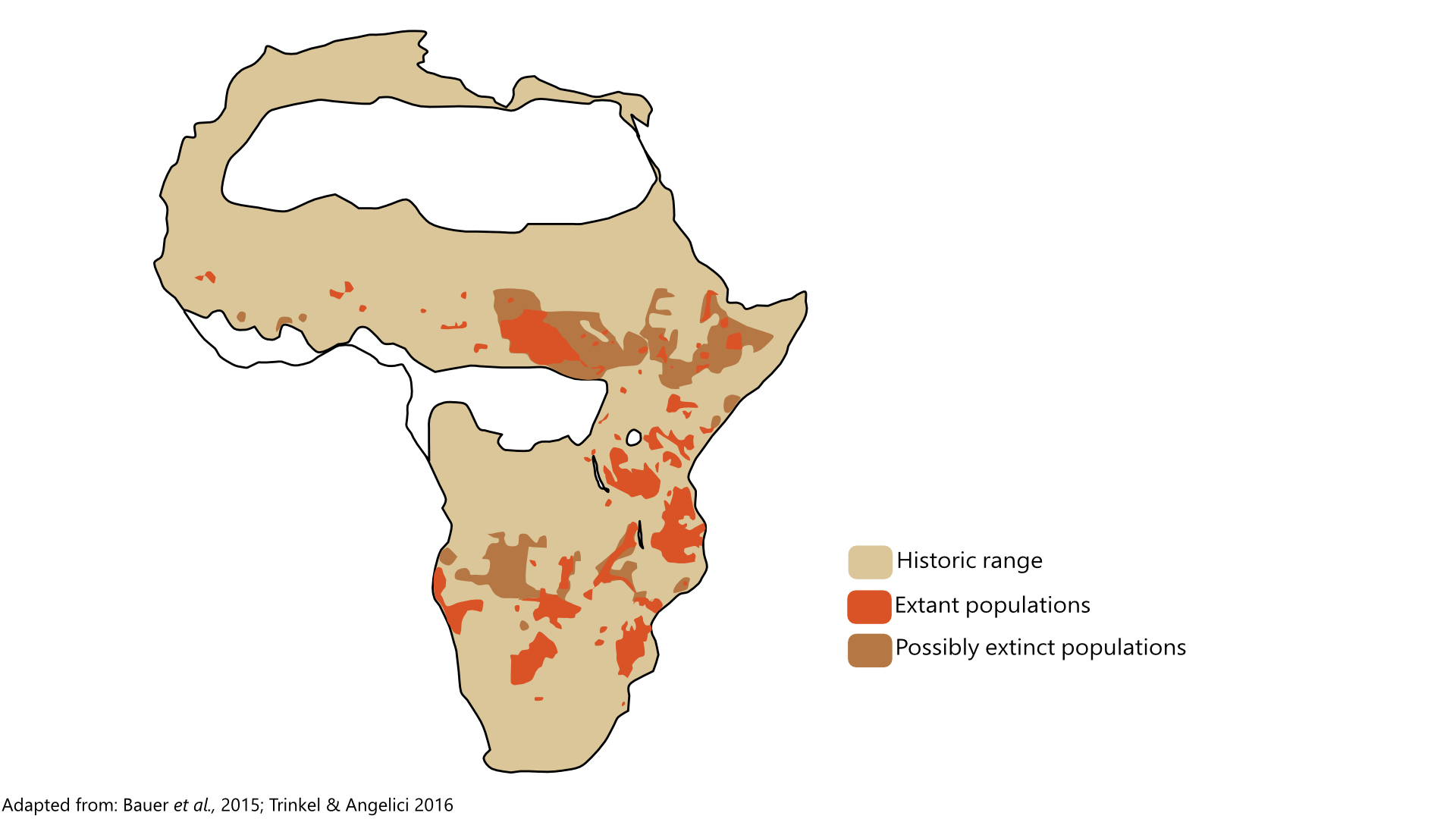
The areas where today’s lion populations are found (red) are merely a fraction of the original distribution zone (light brown). In many other areas (dark brown) the African lion is presumably already extinct. (Figure: © LINC)
The first step involves tracking the movements of individual lions. Machine learning applications handle this part: They can recognize and identify particular animals based on certain facial features, such as eye shape. But why rely on self-learning algorithms here? Wouldn’t it be easier to simply program an algorithm with all the information needed to identify the individual lions?
Think about your closest friends for a moment. It is probably not difficult for you to recognize their faces. However, if you had to put into words exactly how you tell them apart, you would probably realize fairly quickly that your descriptive skills are limited – as will certainly be the case if you include your entire circle of acquaintances in this mental exercise. The same problem arises with the leonine faces in LINC. To put it in different terms: how can a cognitive ability that cannot be fully expressed in words – the ability to recognize faces – be translated into a language that computers can understand?
The answer is disappointing: it is simply not possible, because humans lack the requisite observational powers and linguistic means. That is precisely why the plan is for the computer system to learn to make these distinctions.
In LINC, sample images of particular lions are first input into the computer. This is painstaking manual work; researchers photograph individual lions from various angles, give each animal a number, and annotate each photo accordingly. These sample images are then entered into the machine learning application. Initially, the algorithm decides purely at random whether it recognizes the same animal in two images or whether these photos represent two different lions. It subsequently compares this decision with the correct solution. The system repeats this thousands of times with myriad different images, each time increasing its experience and thus boosting its hit rate.
Step by step, the algorithm thus establishes the categories that it uses to identify individual lions and grows more and more reliable. Like a baby that gradually comes to recognize its relatives’ faces, the application learns to recognize patterns and laws with no need for programmers to identify each of the features used in categorization in order to incorporate these into the algorithm.
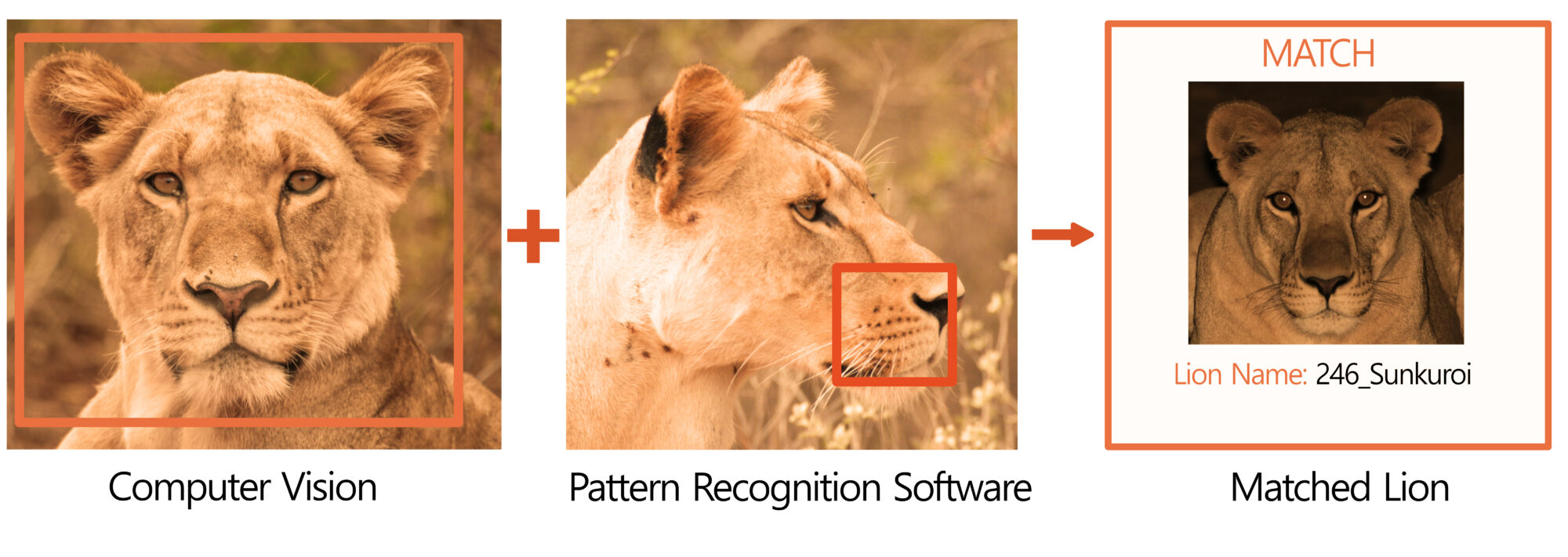
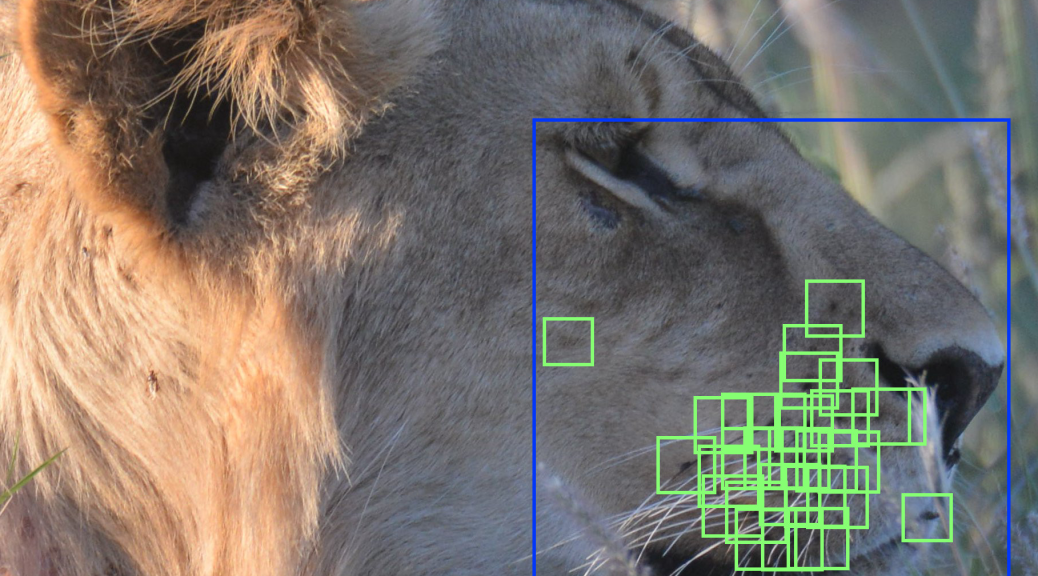
In addition to the facial recognition software, LINC compares the whiskers’ pattern for a match. The comparison process, which is similar to that used for fingerprints, improves identification accuracy. (Figure: © LINC)
Other projects involved in Triggered by Motion use machine learning in similar ways. In Botswana, for example, Gabriele Cozzi’s team identifies African wild dogs based on their fur patterns; in South Korea, Choi Myung-Ae and her team are developing an algorithm that categorizes and counts cranes by species, and the Ibex Project in Italy’s Gran Paradiso National Park is fine-tuning an AI system that can recognize individual ibex.
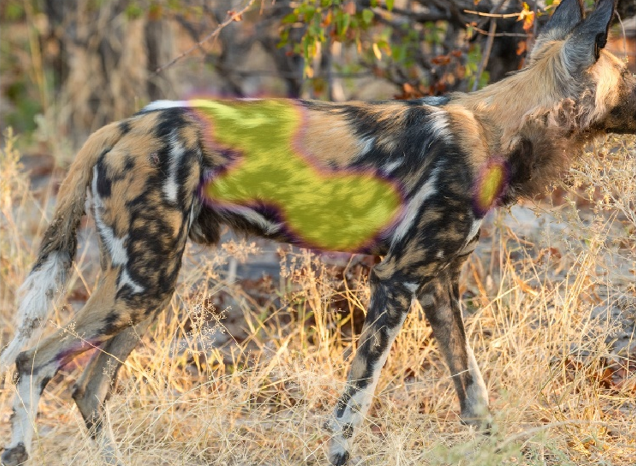
This wild dog could be identified thanks to its distinctive fur patterning. The algorithm first locates the animal in the image and then matches the fur pattern with a database. (Photo: African Carnivore Wildbook / Botswana Predator Conservation Program)
The algorithms are not perfect: if the wild dogs have been rolling in the mud, for example, or if cranes are too far away from the camera trap on foggy days, the systems struggle to record the animals’ details correctly. Nevertheless, machine learning is enormously helpful in research and looks set to grow more important in the future. By connecting researchers, network forums such as LINC and Triggered by Motion help foster technological advances.
Project team:
Lead: Dr. Katharina Weikl, Graduate Campus, UZH
Assistance: Manuel Kaufmann, Graduate Campus, UZH
Project management: Leila Girschweiler (until August 2021), Anne-Christine Schindler (since September 2021), Graduate Campus, UZH
Computational biology: Laurens Bohlen, Graduate Campus, UZH
Pavilion design: Dino Rossi, Impact Acoustic
CNC Operator: Dimitri Zehnder, Impact Acoustic
Mentor: Prof. Dr. Ulrike Müller-Böker, Graduate Campus, UZH
Scientific advisor: Prof. Dr. Daniel Wegmann, University of Fribourg
Editing: Jan-David Bolt, Vanessa Mazanik, Lars Mulle, Vanja Tognola, Hadrami Yurdagün
Sound Design: Lars Mulle
Image editing (postcards): Sebastian Lendemann
We'd like to thank Impact Acoustic for the production of the pavilion out of materials made with recycled PET.
Research partners and other collaborators:
Africa
Kuruman River Reserve, South Africa: Marta Manser, Brigitte Spillmann, University of Zurich, Zoe Turner, Kalahari Research Centre
Lewa Wildlife Conservancy, Kenya: Dominic Maringa, Eunice Kamau, Timothy Kaaria, Lewa Wildlife Conservancy, Martin Bauert, Zurich Zoo
Moremi Game Reserve, Botswana: Gabriele Cozzi, University of Zurich, Megan Claase, Peter Apps, Botswana Predator Conservation
Asia
Chennai, India: Susy Varughese, Vivek Puliyeri, IIT Madras
Cheorwon, South Korea: Choi Myung-Ae, Center for Anthropocene Studies, KAIST
Kastamonu, Turkey: Anil Soyumert, Alper Ertürk, University of Kastamonu, Dilşad Dağtekin, Arpat Özgül, University of Zurich
Seoul, South Korea: Kim Gitae, Citizen Scientist
Shanghai, China: Li Bicheng, Shanghai Natural History Museum (Shanghai Science and Technology Museum)
Europe
Cerova, Serbia: Mihailo Stojanovic, Citizen Scientist
Engadin, Switzerland: Hans Lozza, Swiss National Park
Fanel, Switzerland: Stefan Suter, WLS.CH / Zurich University of Applied Sciences
Gran Paradiso National Park, Italy: Alberto Peracino, Parco Nazionale Gran Paradiso, Alice Brambilla, University of Zurich
Zürich, Switzerland: Conny Hürzeler, Citizen Scientist, Madeleine Geiger, StadtWildTiere Zürich
North America
Bolinas, USA: Jeff Labovitz, Suzan Pace, Citizen Scientists
Palo Alto, USA: Bill Leikam, Urban Wildlife Research Project
Rolling WI, USA: Blayne Zeise, Jennifer Stenglein, Snapshot Wisconsin / USFWS Pittman-Robertson Wildlife Restoration Program
San José, USA: Yiwei Wang, Dan Wenny, SFBBO Coyote Creek Field Station
Oceania
Oamaru, New Zealand: Philippa Agnew, Oamaru Blue Penguin Colony
South America
Pedregulho, Brazil: Rita de Cassia Bianchi, Rômulo Theodoro Costa, São Paulo State University
Rio de Janeiro, Brazil: Natalie Olifiers, Universidade Veiga de Almeida
The international network was developed with support of: swissnex San Francisco, swissnex Brazil, swissnex Boston, swissnex China, swissnex India, Swiss Science & Technology Office Seoul and the Swiss embassy in Nairobi.